When U.S. Navy sailors were captured in the Persian Gulf in 2016, many believed the event was caused in part by Iran’s ‘spoofing’ or falsifying U.S. satellite signals.
With spoofing, jamming, and other recent incidents, adversaries have demonstrated their rising sophistication and intent to disrupt space systems. U.S. military leaders have been strongly vocal about the threats to space capabilities, heightening the urgency and need for more advanced Space Situational Awareness (SSA).
RF monitoring data has long been used to manage signal interference issues; however, RF monitoring can do much more on the SSA front to identify the behavior and performance of the satellites themselves. By leveraging RF data — the information about satellite signals — SSA can better detect, characterize, and attribute threatening space activities. With space evolving to a warfighting realm, RF data can effectively fill the gaps in traditional SSA sensors to provide more timely, thorough, and predictive awareness.
Expanding Beyond Traditional SSA
SSA provides the foundation for managing and maintaining space dominance, from de-conflicting space traffic, to protecting the space assets that are essential to U.S. commercial and national security capabilities.
These SSA functions include the ability to discover, track, and maintain the custody of space objects and events. For example, to detect the risk of high-potential conjunctions as space becomes more cluttered. Further, in an adversarial space environment SSA must be able distinguish and recognize objects, their mission types, and infer whether their intent is benign or malicious. Just as in the ground, sea, and air domains, the awareness or ability to understand space is predicated on completeness of information.
To date, SSA has relied on two main sensor types for data; electro-optical sensors (telescopes), and radar. While each is effective in their own right, they also possess gaps which create blindspots.
For example, few radar systems extend to deep space to track geostationary satellites in orbit 22,000 miles above the Earth. While telescopes can image objects in deep space, they can only do so at certain times and conditions; they’re unable to detect objects or maneuvers in the bright light of day, or when obscured by cloud cover.
RF as Unique Data Domain
RF, the medium by which satellites communicate, offers a wealth of information absent from other traditional sensors. Whereas a maneuvering satellite can be beyond the range of radar and hidden from telescopes by weather, its RF transmissions can be monitored day or night, in all weather conditions to detect maneuvers and anomalies. Further, because RF data is about the physics of the satellite signals (not their content), it can be used to interpret a craft’s payload performance and usage, including the type of traffic and mission it supports. Those insights can help determine whether an unexpected maneuver represents the intent of an adversary or is caused by an onboard system failure. These RF capabilities fill the blindspots in existing sensors, while providing additional knowledge.
RF data has supported space operations for years, from monitoring payload performance to detecting and geo-locating interference. This data always held promise for SSA, but wasn’t available at scale due to limited sensor coverage and infrastructure.
Now, with the availability of commercial global monitoring solutions, which can supplement government resources, RF data can be collected for the complete geostationary arc at all times boosting the ability to detect, characterize, and attribute space threats. Combined with advances in data analytics, machine learning, and AI, RF can provide additional insights that elude detection or characterization by traditional sensors. Fused with other data, this offers a more complete and accurate real-time picture of satellites, their activities, and the impact to users of these systems.
Taking strides to advance its space capabilities, the Department of Defense (DoD) launched the Joint Interagency Combined Space Operations Center (JICSpOC) in 2015 to develop, test, and integrate new tactics and techniques to support its space operations. Located at Schriever Air Force Base in Colorado Springs, this fusion hub, recently renamed National Space Defense Center (NSDC) is leveraging commercial RF and EO data in support of the national security space enterprise. In 2018, the NSDC transitioned from experimental to fully operational status.
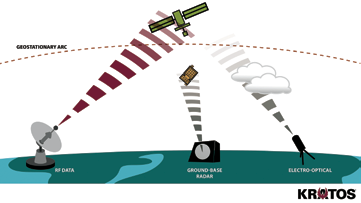
Figure 1. Two main sensor types for SSA data, Radar and Electro-Optical (EO), each offers strengths, but also limitations. RF data can fill gaps to enhance SSA. Image is courtesy of Kratos.
The Source of RF Data
RF data is the information about the signal externals or waveforms from satellite uplinks and downlinks. Monitored and collected for multiple bands and frequencies by a worldwide network of sensors and antennas, this signal data is represented by over a dozen measures. These include: directional RF power, center frequency, signal-to-noise, carrier-to-noise and more. These metrics can be used to determine the Location, Health, Usage and Attribution of space assets — all of which support the functional requirements of SSA.
How RF Detects Maneuvers and Location
As a satellite moves, its RF signals exhibit a small Doppler shift in its Center Frequency (please see Figure 2). This indicates the satellite’s positional change and movement. By persistently monitoring these frequency shifts, satellite maneuvers can be detected as they occur in real-time, maintaining chain of custody for systems of interest that can evade other sensors. These RF location updates, combined and correlated with optical and radar sensor data, better support conjunction assessment for satellite collision avoidance and safety of flight.
By applying machine learning and pattern recognition algorithms to RF data, the normal ‘patterns of life’ for space objects can be established. Any deviations or anomalies from these patterns can be detected and alerted for more pre-emptive threat awareness and space traffic management.
RF to Understand Payload Health and Usage
RF data provides numerous insights on payload performance and status, that is: when systems are operating nominally, when they’ve changed or degraded and to what degree. (See Figure 3.) For example, slow, gradual variations in normal measures might indicate equipment wear or changes in user requirements. More sudden or severe RF signal deviations, on the other hand, may uncover a payload system failure, jamming or a directed energy attack.
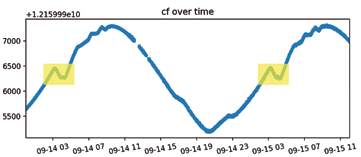
Figure 2. Doppler shifts detected in the RF signal of a satellite indicate its movement at specific times. Image is courtesy of Kratos.
This ability to detect payload irregularities and their origin accelerates response and resolution. For example, operators would know whether to re-route traffic due to an equipment issue, or to focus on geo-locating an unauthorized broadcaster to eliminate interference.
Data about transponder and communication links also provides an understanding of the amount, type, and nature of payload traffic. For example, RF signal characterization can determine whether an asset’s transmission is supporting video conferencing or potentially hostile UAV operations, and whether a large spike in a certain traffic type may be an indication or warning.
These RF insights also provide battle damage assessment. This helps identify the capabilities of friendly, neutral, and enemy space systems, including the impact and effect of offensive or counter-operations. For example, detecting a change in a transponder’s signal might reveal its reduced traffic-carrying capacity, and as a consequence, the satellite’s reduced mission capabilities.
RF to Illuminate Attribution
RF monitoring immediately detects any disruption to satellite signals whether originating in orbit or on the ground, whether intentional or inadvertent.
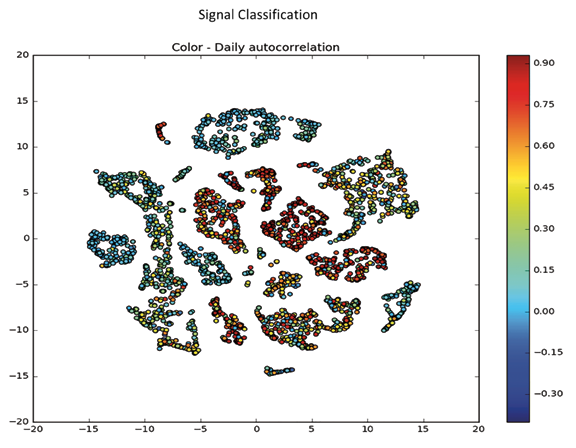
Figure 3. Clustering algorithms classify the types of RF traffic from a satellite. The color-coded bubbles represent the numerous channels and types of traffic being carried. Image is courtesy of Kratos.
There are a variety of threats to satellites, yet unintentional interference is the most common. RF techniques can characterize and identify interfering activities, whether due to the congestion of multiplying VSAT terminals, misconfigured gateways, intruding signals from neighboring satellites, the “blue’ or friendly interference that can often happen in naval fleets or on the battlefield. RF can also evaluate the frequency ‘noise’ of an orbital slot before a satellite moves to it, so it can be prepared to avoid ensuing interference.
RF techniques are becoming increasingly more accurate, geo-locating interference to within a few kilometers. With additional signal characterization, the type of modem can be fingerprinted and the carrier identified to help narrow attribution to an entity or organization. With inadvertent interference, resolution often entails simple coordination with an owner or operator to reconfigure an antenna or terminal; whereas intentional jamming can employ RF capabilities to mitigate and cancel out the interfering beam.
Adding Value with Data Science
Just as RF methods have been honed over the years to support satellite mission performance and interference detection, new RF techniques are being developed to exploit its value for SSA. With the correct RF analytics, machine learning, and AI tools, personnel can characterize the attributes of satellites, predict the maneuvers or actions they may undertake, and discern intentions. For example, the automated classification of bandwidth use, transmission type, and timing can help identify satellite modems, payload activities, and attribute behavior.
This machine-speed data collection and analysis supports more predictive warning, extending lead times and knowledge for appropriate response. For example, early detection of satellite interference from RF, correlated with other events, such as cyber disruptions and upticks in social media activity, could indicate precursors of hostile actions. Fusing RF analytics with other data sources can provide decision-makers the information needed to get ahead of mission threats, such as triggering maneuvers to avert
a collision.
SSA for a New Space Era
Space systems are exposed to a growing array of threats; from potential collisions and spectrum interference as more satellites are launched, to adversaries turning over new techniques to disrupt and disable space operations. These challenges have placed urgency on advancing the timely, complete picture required of SSA.
The addition of the RF domain adds new dimensions to SSA, filling in gaps and advancing capabilities beyond what’s possible with traditional sensors and data. The unique attributes of RF data to detect, locate, and characterize space systems supports the more predictive and pre-emptive decision-making required for this
new era.
For more information about RF data and its application to Space Situational Awareness, read the white paper located at: www.KratosDefense.com/SSA
Greg Caicedo, the Vice President of Kratos Network & Data Solutions, is responsible for EMI Spectral Services, SSA Solutions, RF Data Products, and Network Product Solutions for Kratos, serving both commercial and government markets. His 25+ years of experience in program management, operations, and leadership positions have supported a range of space programs and missions, including National Space, Launch Vehicles, Space Situational Awareness and SATCOM missions. A retired Air Force Officer with 20 years of service, he was involved in Space Acquisition, Operations, and Policy for National and AF programs.
What’s Required to Convert RF data into SSA Intelligence?
Kratos operates the most extensive, fully-integrated, commercial global RF monitoring network, which has been helping government and commercial customers detect and resolve RF spectrum challenges for years. This custom developed infrastructure includes:
A global sensor network: Consisting of 21 worldwide RF monitoring sites, hosting more than 80 fixed and steerable sensors and antennas in C- and Ku-bands, as well as X-, Ka-, L- and S-bands. Using state-of-the-art sensors, the network is tuned for high-speed, accurate RF signal collection and measurement.
A well-staffed, state-of-the-art RF operations center: The central hub for monitoring and integrating raw RF data from its global sensor network. The NOC incorporates automated workflows, tasking, and visualization that support Kratos’ workforce skilled in RF spectrum management and SSA techniques.
Data Science and algorithms: Kratos’ advanced analytics and AI tools process RF data for real-time SSA awareness, predictive insights, historical trending, and patterns of life. Fused and correlated with data from optical, radar, terrestrial and space-based sensors, this provides more timely, accurate, and complete SSA.